Artificial Intelligence in Cancer Research: A Game Changer for Healthcare
But it’s been possible to make this progress because of the field of intelligence (AI), which has made it possible to pursue research on cancer. AI could change how we diagnose, treat, and prevent forms of cancer. With this evolving field growing, scientists and healthcare professionals are now integrating AI as a tool to tackle the minefield of challenges created by this appalling disease.
Image analysis for detection and diagnosis using AI has also found one of the more interesting applications in cancer research. A good example: the National Cancer Institute researchers have developed a learning algorithm that automates the process of identifying precancerous cervical lesions on digital images. In addition to improving screening accuracy, this breakthrough also has lifesaving implications: it means that cancer can be found at an earlier stage.
As you can imagine, AI is used in precision medicine, specifically working their way through datasets with multiple variables to personalize treatments for individual patients. This tailored approach to cancer care has the potential to change cancer care. However, it will significantly improve patient outcomes.

The Fundamentals of AI in Cancer Research
Understanding Artificial Intelligence
Refers to the development of computer systems that form intelligent systems and can perform tasks that normally require intelligence. Learning, problem solving, reasoning, and pattern recognition are among these tasks. In recent times, the intelligence (AI) field has come a long way, thanks to the advances in high-performance computing and the abundance of data available. Across all domains, including cancer research, two important areas, machine learning (ML) and deep learning (DL), have seen increasing importance.
Machine learning is the use of algorithms that learn from data, allowing computers to make predictions or decisions based on the data they receive. Machine learning in general, and deep learning in particular, are areas of machine learning that try to reproduce similar layers of neural network functions to process and recognize patterns similar to how data passes through and is processed by the human brain.
The Role of AI in the Medical Field
The potential is great in the AI field; it provides great benefits for the whole range of. In cancer research, specifically, AI can play a role in:
- Cancer Detection: Our ability to analyze medical imaging data collected from patients undergoing X-ray CT. and MRI scans has allowed us to use AI-driven tools to find, locate, and classify cancerous tumors. The early detection could greatly increase the rates for successful treatment.
- Diagnosis: AI techniques can process such amounts of data as patient records and genetic information sets and identify patterns, which lead to cancer development. It helps scientists pinpoint things that increase a person’s risk of contracting cancer and better screening methods. It thus results in diagnoses and personalized treatment plans.
- Genomics Analysis: This allows AI to look through a sea of data to find certain gene mutations linked to cancer. It helps us understand how cancer develops and also to design targeted therapies.
- Treatment Planning: Patients’ medical history, cancer stage, genetic composition, and a variety of other factors are analyzed by AI, and it decides on the treatment. It is tailoring treatment strategies to patients, which could increase the outcome.
- Drug Development: AI analyzes gene, protein, and other molecules connections to assist in developing targeted cancer treatments. The methodology significantly decreases the time and cost of drug discovery methods.
Participating in the frontier of AI applied to cancer research not only further educates you but also drives toward novel discoveries to combat, detect, and treat cancer.
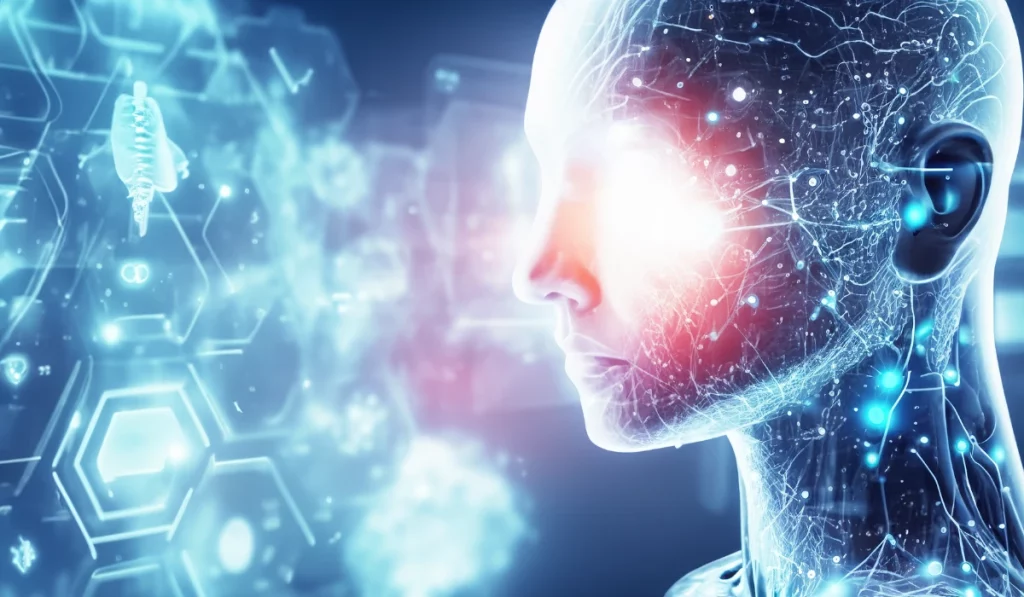
Evolution of AI for Cancer Detection and Diagnosis
From Screening to Precise Diagnosis
AI has moved cancer detection from screening techniques to more accurate diagnosis techniques in a domain. In the first instance, sensitivity and specificity screening based on mammograms and routine checkups was the method for early detection. But, over the years, we’ve seen the invention of methods to identify and classify cancer, due to the development of AI algorithms and the improved computing power. The progress created an opportunity to advance approaches to precision medicine.
An advantage of using AI-based models for cancer diagnosis is that they are able to analyze large amounts of data to detect patterns that aren’t easy to spot by observers. On the path towards medicine, they are expected to use AI-based models to decide what cancers should be treated based on the individual characteristics of the tumor and its genetic profile.
AI in Histology and Cancer Imaging
It is fair to say that AI has significantly revolutionized histology and medical imaging. The identification of indicators of cancer in samples that were previously processed visually by highly skilled pathologists under microscopes has been made much easier. AI algorithms today are used to recognize patterns, identify biomarkers, and classify types of cancer.
AI is changing the way we do MRI scans and biopsies in imaging, beyond radiology. Examples of AI inclusion in an exam such as a mammogram or an MRI scan have been integrated, which translate to accurate screening and detection of cancer. These AI-driven algorithms, being able to analyze the data, can identify the subtle patterns and features in amounts of data that healthcare professionals would not see. In turn, cancer detection becomes more accurate.
Challenges of Using AI in Cancer Detection
Though there has been potential use of AI in cancer detection and diagnosis, there are also hurdles. For example, the success of AI models hinges heavily on the quality and quantity of data that was supplied to the models while they developed and were trained. The lack of a good representative dataset is caused by variations in cancer types, stages, and patients.
There is yet another challenge regarding standards and rules for construction, validation, and use of AI models. That’s about ensuring that the model learns in such a way that it minimizes the effects of algorithmic biases and optimization to minimize data privacy violations and making that process transparent and interpretable. Moreover, deep integration of AI into healthcare systems requires the cooperative efforts among healthcare professionals, researchers, developers, and regulatory authorities.
Finally, the development of AI for cancer detection and diagnosis has promise to improve cancer outcomes. With this, we could achieve remarkable progress in the implementation of precision medicine and early cancer detection opportunities if these challenges were surmounted and if capabilities afforded by AI technology were harnessed effectively.

AI in Cancer Treatment and Follow-up
AI Role in Drug Discovery and Repurposing
AI in cancer treatment and follow-up plays a role in drug discovery and repurposing. This greatly increases the efficiency as well as the accuracy of the process. Against the backdrop of cancer, AI algorithms analyze data and can predict what type of drug compounds would be most effective at treating certain types of cancer. They can also decide which drug combinations would prove efficacious. It speeds up the development of therapies. Finds existing drugs that can be repurposed to treat prostate, breast, lung, and colon cancer.
Similarly, machine learning techniques were used to predict side effects and levels of toxicity of drugs. It helps researchers determine which drugs work for cancer patients.
Precision Oncology and Therapeutic Targets
Precision oncology sources both identification of targets and development of personalized treatment plans in patients, based on patients genetic profiles and tumor characteristics. By analyzing profiles of cancer cells, AI algorithms can determine which treatment best suits each patient, avoid remission, and reduce side effects.
Researchers can with the assistance of AI. Therapeutic targets—genes or proteins that are essential for cancer cell survival and proliferation. This important information is vital for developing therapies that are discriminating against cancer cells and spare tissue. AI can also be used to develop ultra-risk treatment plans for cancers such as prostate, breast, and lung cancer. It also identifies subtypes. The ability to predict patients’ response to treatment.
AI Assisting in Treatment Response Assessment
AI has a significant contribution to make towards predicting treatment responses. This greatly facilitates clinicians to have accuracy and speed in monitoring progress. The algorithms that make up AI can help provide real-time feedback on treatment responses by analyzing patterns in medical imaging data. It also allows changing treatment plans if necessary.
In practice, people use AI-based systems to monitor tumor size, shape, and volume over time. And these changes are consequences of the efficacy of a cancer therapy. Additionally, AI can help predict the chance of recurrences of cancer. It helps both clinicians and patients make informed decisions both before and throughout the cancer journey.
It should be noted finally that AI is currently changing the face of both cancer research and treatment as it can accelerate drug discovery, as well as repurposing efforts, to assist in ‘tailoring’ therapy options to the patient’s specific needs, as well as in assessing treatment response. This is a powerful technology that can change the landscape of cancer care, and in a good way, by improving outcomes and revolutionizing where we are with oncology today.

Cancer Prediction and Prognosis
AI in Predicting Cancer Prognosis
In other words, artificial intelligence is used to predict the prognosis of cancer, assisting the professionals during making the choice that could be life or death. Massachusetts General Hospital datasets can be analyzed by advanced AI tools to forecast breast cancer cases, for example. With the help of AI-based techniques like radiomics, the expected cancer outcomes can be predicted as well as the improved treatment strategies can be developed.
In addition, AI methods can look at tumor pathology images to identify gene mutations instead of through traditional genomic sequencing. The result is that this accelerates the process and can also help identify those treatments that will work best for patients.
Addressing Cancer Recurrence
The prognosis of cancer includes the aspect of dealing with recurrence. Prediction of the probability of cancer recurrence by analyzing survival data and generating treatment plans can be aided by AI. Such care can boost comprehensive care and the chances of long-term survival of the patients.
One example would be using AI to enhance cancer research, such as putting the power of AI to good use to bridge a challenge such as predicting a glioma tumor’s future repeat. By integrating, the researchers gain some understanding of deadly diseases. They can help develop new anticancer therapies or guide the development of new anticancer therapies to lower the possibility of recurrence.
In a nutshell, the work of AI in the field of cancer prediction and prognosis has been promising. It can predict cancer whether it will come back or not. Provide treatment plans. AI is already revolutionizing cancer care and will continue to do so moving forward through advancements and collaboration with researchers.
The Limitations and Challenges
Ethical Considerations in AI Implementation
Implementing intelligence in cancer research is a serious business to consider. The biggest part is protecting patients’ data to ensure privacy and security of the same. It is necessary to comply with data protection laws and to lay out the barrier from which any access to sensitive information will be prevented.
To another ethical issue, oncologists are concerned here—of Albert Einstein’s, if you can afford it, bias in AI algorithms that could lead to interface types for cancer diagnosis and treatment. One way to solve this problem is to develop an AI model based on representative data without discriminating on race, gender, age, or such other factors. Transparency and explainability of AI algorithms are also important to permit professionals to understand and trust the recommendations made by AI.
Addressing the Pitfalls in AI Deployment
Implementing AI in cancer research requires solving the problems and limitations in it. Some of these hurdles include:
- Data quality and availability: The training and validation of AI models require acquiring high-quality datasets that are representative and correctly labeled. Accurate, up-to-date data with labeling is difficult to achieve. Furthermore, the development of AI models in cancer research heavily depends on data sharing and collaboration within different research institutions.
- Algorithm development and validation: Creating AI algorithms that are accurate and generalizable for oncology is a tough task that involves not only a deep dive into oncology but also one into artificial intelligence. Additionally, this model’s validity needs to be tested using independent datasets to ensure the performance predicted and safe deployment in the real world.
- Integration into clinical practice: For AI to work in cancer research and practice effectively, those building the AI need to work closely with oncologists and other medical personnel. In this collaboration, we work on adapting workflows and bringing the results from the insights derived from AI to professionals, as it supports applying them into their practice.
- Addressing regulatory and legal issues: Cancer research moves quickly with AI. Standards and guidelines will be set up now by regulatory bodies to ensure that AI-driven tools are always safe, effective, and ethical. Successfully implementing these requirements in AI applications for cancer research is part of navigating.
By accepting the hurdles and limits of using AI for cancer research, we will apply effort to address these issues to the best possible extent to leverage the AI for fighting cancer.

Future Perspectives of AI in Cancer Research
The Role of High-Performance Computing
With each advancing step in intelligence (AI) research, so too does its use in cancer research. In this process, high-performance computing (HPC) is a part of this process, enabling analysis and interpretation of large-scale datasets produced in the field of oncology. HPC AI can do at this level. We provide analysis of amounts of genomic, transcriptomic, and imaging data, which add to our understanding of the highly complex molecular processes that fuel cancer.
In addition to accelerating research, HPC helps reveal targets and the development of personalized medicine for the treatment of cancer patients. With the combination of AI and HPC, we can make accurate predictions of drug responses or drug treatment outcomes. Together, this integration provides information for providers to make decisions and provide personalized cancer therapies.
Pioneering AIs Future in Cancer Research through Coding
That’s why, down the line, coding will be crucial to unlocking the potential of AI in cancer research. Factors that will drive advancements in the field include adopting new coding practices and innovative deep learning architectures.
- Collaborative platforms: If collaboration is fostered through platforms, then making progress is necessary. By jointly enabling teamwork between professionals in different fields, we together will contribute to innovating cancer research. This helps us streamline the development of AI-powered tools and models that will increase their accuracy and efficiency to understand cancer data by coordinating the efforts of experts in oncology, computer science, and bioinformatics.
- Inclusion of rare cancers: Understandably, to move forward with cancer research that uses AI is important to understand the molecular basis of all types of cancer, including those that are rare. It expands the scope of AI analysis from these represented cancers to improve outcomes and establish personalized treatments.
- Human intelligence augmentation: With AI’s tools and support for cancer research, the work being done isn’t lessened because of the work human’s intellect can (and will) provide. Instead, we should be aiming to help you become better oncology researchers, clinicians, and decision-makers, giving you effectiveness and knowledge to your endeavors.
What’s coming next is further convergence of AI high-performance computing and advanced coding practices that will continue to redefine cancer research and personalized clinical care. While continuing to deliver a touch throughout the journey, we create space for revolutionizing cancer treatment strategies by engraining collaboration and technology integration.